Investing in Enterprise AI Startups: Why I’m Skeptical and Staying Away
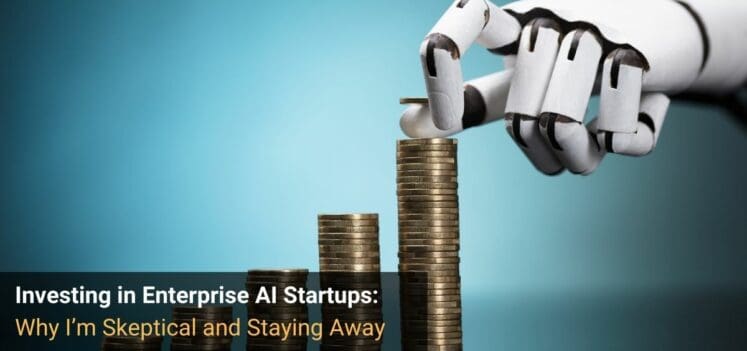
Ever since ChatGPT burst onto the scene in November 2022, there has been overwhelming hype about all things artificial intelligence (AI).
That hype has led to vast funding for AI startups: Over $35 billion in the first half of 2024 alone, which was around 21% of global VC funding for the period.
But doubts have also emerged about the business model, signaled by Sequoia’s release of its “$600 billion question” analysis (i.e., where is the extra revenue from hundreds of billions spent on GPUs and data centers?).
Then there was the Goldman Sachs AI report, which took a measured tone and presented both sides of the debate.
Meanwhile, the other big questions about whether AI will kill all jobs / remake human civilization persist.
These are interesting debates, but I want to examine a much narrower topic here and explain my skepticism of one category: VC-backed startups attempting to sell generative AI products (agents, workflow automation, customized chatbots, etc.) to enterprise customers.
I’ll start with the short version and then explain each factor in more detail below:
- The TL;DR Version of My Skepticism Toward (Enterprise) AI Startups
- Do AI Startups Benefit from AI?
- Most AI Startups Are Service Businesses, Not Software Businesses
- Most Startups Do Not Know How to Be Service Businesses
- AI is Mostly a New Feature, Not a Product
- Big Tech Companies Are On the Ball This Time Around
- Startup AI Deals Could Hurt VC Investors
- And Why I Could Be Wrong About AI Startups
- The Biggest Misunderstanding About AI Startups
- For Further Learning
The TL;DR Version of My Skepticism Toward (Enterprise) AI Startups
- Most of the benefits of generative AI will flow to individuals and small businesses using the products and Big Tech and consulting firms training / implementing models or providing the infrastructure.
- Most enterprise AI startups are more like service businesses than traditional software / SaaS companies in terms of margins and scalability (i.e., don’t expect the “Rule of 40” here). And most startups are terrible at delivering services to large enterprises.
- Most AI startups are not doing anything defensible because they lack proprietary data, and the training data is the key value driver for most AI models, products, and features.
- AI is more of an additional feature for existing products / services than a completely new “product category” (with some exceptions in the physical realm and for consumer products).
- In previous tech waves, such as the Internet in the 1990s and mobile/smartphones in the 2000s, Big Tech companies were slow to respond and adapt, but they are in a much stronger position today and have already benefited disproportionately.
- If you look at AI startup exits so far, many have been structured as licensing / acquihire transactions in which the big company does not technically “buy” the startup. This practice could reduce exit values, dampen returns for VC investors, and disadvantage rank-and-file employees.
Do AI Startups Benefit from AI?
Most peoples’ immediate, gut-level reaction to these arguments goes like this:
“But ChatGPT or AI Feature X is so useful! It saves me time and lets me get more work done.”
I agree!
Small businesses and individuals benefit from generative AI because they are time-constrained and can’t necessarily afford more employees or contractors, so it lets them accomplish more with less.
However, the specific question here is who profits from these features, not which users benefit the most.
At this stage, the answer appears to be “Big Tech companies and consulting firms ” because they’re in one of the following categories:
- They sell the hardware and infrastructure (Nvidia).
- They have massive amounts of data, the resources to train models with it, and the ability to deploy AI features to mass-market products (Microsoft, Google, Facebook).
- They sell expensive consulting services for AI implementation (BCG, McKinsey, Accenture, etc.).
In my view, VC-backed startups are stuck in no man’s land.
Sure, they can develop products more quickly with tools like CoPilot and GPT-4, so they benefit from generative AI in that sense.
But they have no advantages in training data or sales & marketing, which are critical if they want to develop and sell valuable products to other companies.
Simple ChatGPT wrappers have their uses, but they’re not defensible, and I don’t think any large B2B companies will be based on them.
Most AI Startups Are Service Businesses, Not Software Businesses
You might be surprised to learn that Accenture has generated $3.6 billion in annualized Generative AI bookings vs. OpenAI’s annualized recurring revenue of $3.4 billion.
But if you understand how Large Language Models (LLMs) work, this is not surprising.
In short, it is difficult to build a “one-size-fits-all” product because each enterprise has different needs linked to its own workflows and proprietary data.
Each customer requires a different model to be trained and customized based on this data, and this customization requires computing time and human labor.
And once v1 is done, it must be tweaked, updated, and re-trained over time.
There’s nothing “wrong” with this business model, but it’s much closer to an agency or professional services company than a traditional enterprise software startup.
Many VCs seem to assume they’ll get SaaS revenue multiples of 5 – 10x if/when these companies go public, but I believe services-like multiples are much more likely.
For reference, Accenture currently trades at ~3x revenue, IBM trades at 3 – 4x revenue, and Cognizant trades at ~2x revenue.
Again, these are all perfectly viable and profitable businesses, but they don’t have the same margin or valuation profile as true SaaS companies.
Most Startups Do Not Know How to Be Service Businesses
OK, there is one specific problem with being a service business besides the lower margins and valuation multiples: Most startups and growth-stage companies are bad at it.
Success stories such as Palantir and Red Hat exist, but they are more like exceptions that prove the rule.
The issue is that large enterprises have heaps of compliance, safety, and privacy requirements, and any product/service they buy must be certified against all of them.
There’s more to it than that, which this Hacker News thread details, but in short, selling to large companies is much harder and more resource-intensive.
And other large companies, like Big Tech, inherently have an advantage in this sales process because they understand the requirements and have the required resources.
AI is Mostly a New Feature, Not a Product
By now, you’ve probably seen “AI features” added to countless products, from Word to Gmail to Photoshop to Google Search.
We can debate their usefulness all day, but the point is that large companies can easily add these features to existing products used by millions or billions of people.
One difference in this wave vs. earlier ones, such as smartphones and mobile apps, is that previous platforms made new types of products possible.
The perfect example is Uber.
Microsoft could not have “added” Uber to Windows or Office, and Google could not have “added” Uber to Search because it requires real-time location data via GPS.
But so far, most enterprise AI startups have been launching services that could be add-on features for existing products (voice assistants, document search, coding assistants, cybersecurity, etc.).
Startups targeting enterprise customers need to make the case that they’re offering something that won’t be in the next version of Slack, Acrobat, Photoshop, Word, Outlook, or GitHub.
For this reason, I’m more optimistic about hardware-related AI startups doing something not possible currently in the physical world.
Big Tech Companies Are On the Ball This Time Around
If you look at the boom in startup activity right after the 2008 financial crisis, most Big Tech companies were fairly cautious.
Following the subprime crisis and bank bailouts, one knew exactly what was happening in the broader economy, and companies like Apple, Google, Microsoft, and Amazon kept investing but focused on smaller deals and IP acquisitions.
They were not completely revamping their business models.
This explains how companies like Stripe beat incumbents like PayPal (which was a part of eBay back then) and how companies like Uber, Airbnb, and WhatsApp succeeded.
By the time Big Tech noticed them, they were so big they had to be independent (Uber and Airbnb), or they commanded huge prices in acquisitions (WhatsApp and Slack).
But as of 2024, Big Tech is essentially driving the entire S&P 500 and has had incredible revenue growth over the past ~15 years (Google grew over 10x, and Facebook grew by over 100x).
These companies are far more powerful and influential today, which means they have far more resources to invest in AI features and will not “ignore” promising startups.
OpenAI is a possible exception, but since Microsoft owns ~49% of its for-profit arm, I view it as more of a Microsoft extension.
Startup AI Deals Could Hurt VC Investors
If you look at the structure of many AI startup exits so far, they’ve worked like this:
- Big Tech Company pays a “licensing fee” to the startup, which may or may not repay the VC investors.
- Some of the employees join the Big Tech company.
- The AI startup keeps operating but without the co-founders or key employees.
Google did this with Character.AI, Microsoft did it with Inflection, and Amazon did it with Adept.
Big Tech is doing this to avoid heightened antitrust scrutiny, but this practice potentially hurts both VCs and normal employees.
VCs are less likely to get high exit values if official “sales” do not take place, and at least some employees could easily lose their equity in these deals – which reduces the incentives to join these startups.
Big Tech realizes that many AI startups have no viable business models as independent entities, but they could succeed as add-on features within mass-market products – so they use these deals to acquire talent and features.
And Why I Could Be Wrong About AI Startups
I’ll now add some caveats and disclaimers because my predictions have been wrong before (e.g., Credit Suisse, the markets in 2023 following Silicon Valley Bank, etc.).
I’m 100% certain that additional AI startups will have good outcomes.
I just think the scale of the success will be smaller than what VCs expect and that most of it will happen outside the enterprise sector:
- Companies addressing certain underserved niches could do well, though they won’t reach the scale of Google or Facebook.
- I could see spin-offs from big companies or institutions with tons of valuable, proprietary data also doing well.
- Service/agency businesses can be successful, and there’s no shame in starting or joining one; it’s just that they lack the financial profiles of traditional software or biotech businesses.
- And, of course, AI presents many opportunities for small, cash-cow businesses to succeed because plenty of consumers will pay small monthly amounts for simple AI-based apps for photo generation, outlines, summaries, etc.
The Biggest Misunderstanding About AI Startups
The biggest problem here is that “usefulness” does not necessarily imply “profitable investment.”
Think about how “useful” Facebook is today (ads, spam, high school friends posting stupid political commentary, etc.) vs. how much money it makes.
I don’t think I’ve used Facebook for anything other than occasional Messenger chats since ~2014, but it still prints cash because hundreds of millions of people spend all day online addicted to digital drugs.
So far, much of the output from enterprise AI startups is in the “potentially useful but difficult to monetize independently on a large scale” category.
Maybe that will change over time, but for now, I’m betting on Big Tech and service/consulting firms.
After working to exhaustion/burnout on my current business, I don’t have much desire to start something new.
But if I were starting a new online business today, I would find a way to use generative AI to do more with less.
VCs would not benefit from this, but that’s their problem.
For Further Learning
I recommend the following if you want to learn more and see some of my sources:
- Goldman Sachs – Generative AI: Too Much Spend, Too Little Benefit?
- WSJ – The Big Risk for the Market: Becoming an AI Echo Chamber
- Enterprise AI Trends Substack – Most AI Startups Are Service Businesses Pretending to Be Product Companies
- Sherwood News – Who is Winning the Generative AI War? Accenture
- NY Times – The AI Boom Has an Unlikely Early Winner: Wonky Consultants
- NY Times – The New AI Deal: Buy Everything but the Company
Free Exclusive Report: 57-page guide with the action plan you need to break into investment banking - how to tell your story, network, craft a winning resume, and dominate your interviews